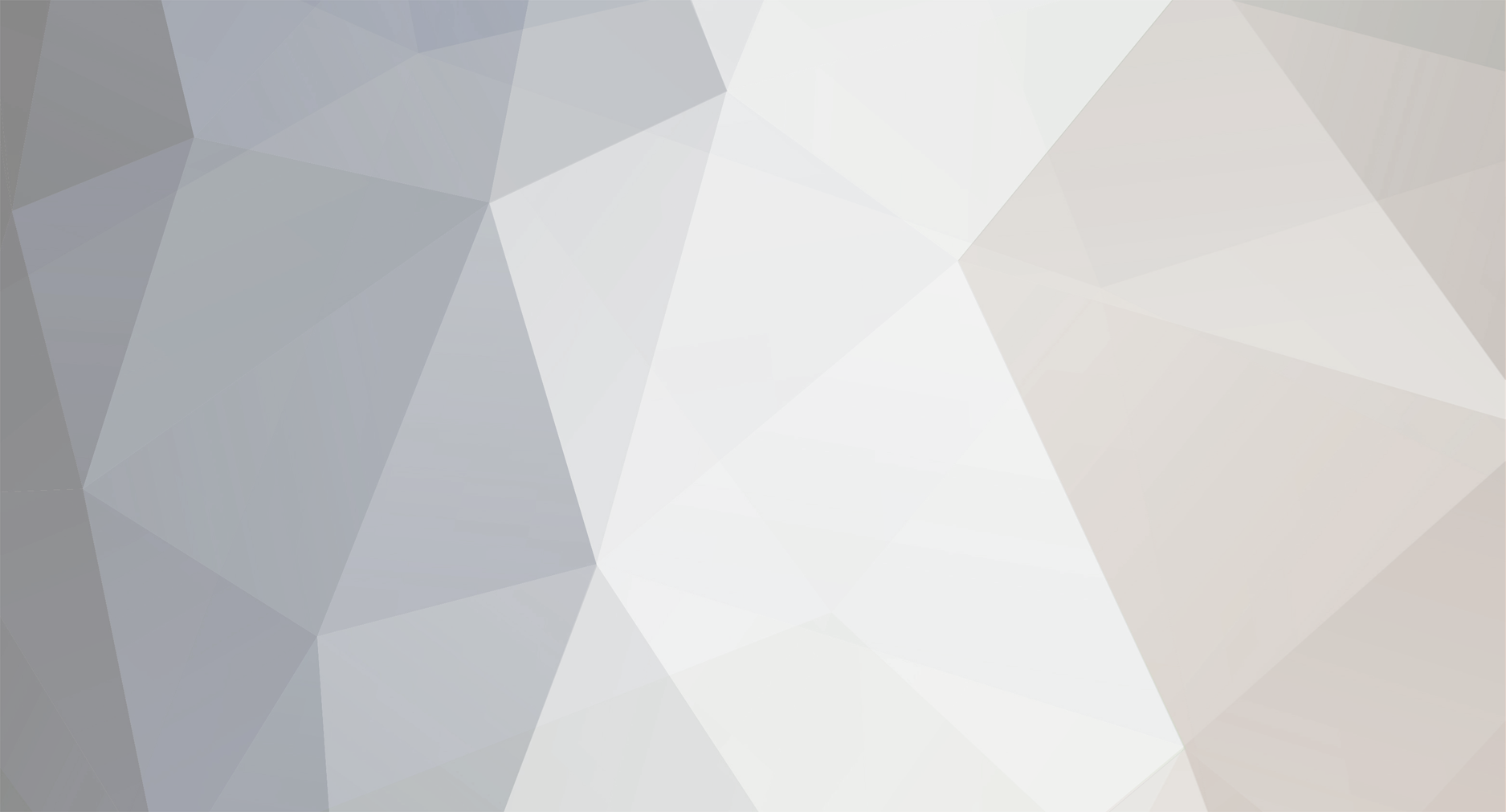
ajreich
Members-
Posts
87 -
Joined
-
Last visited
Everything posted by ajreich
-
January 2024 Weather in the PNW (Part II)
ajreich replied to Meatyorologist's topic in West of the Rockies
Quite the storm this week in the cascades, from the Portland AFD: -
January 2024 Weather in the PNW (Part I)
ajreich replied to Sunriver Snow Zone's topic in West of the Rockies
For sure, but this will likely be improved as training expands to include synthetic meso- and local scale data. Now that the global systems are largely past the proof of concept stage, the finer grained tools are just a matter of time. Exciting stuff! Thanks for all the hard work making this forum what it is -
January 2024 Weather in the PNW (Part I)
ajreich replied to Sunriver Snow Zone's topic in West of the Rockies
Not quite in the same way, since they are by definition probabilistic. The AI models are essentially taking a complex set of atmospheric conditions and turning them into mathematical vectors that can be used to query for similarity - the process of training is itself probabilistic, so you only need run the model once to determine the highest probability outcome for a given set of specific criteria. This is one of the fundamental advances in the field. Ensembles only exist to deal with the inherent stochastic limitations of a deterministic physics model; they are trying to capture the probability distribution, but in a very backwards way. We never known whether the ensemble distribution is representative of reality, or just a distribution of the limitations of the underlying physics packages. AI model solutions generally reflect the probabilities of actual weather conditions occurring, which is why they are/will be so much better than our current model outputs. Add in the simplicity of adding additional data (adding additional vectors without having to bother with physics whackamole), the infinite geographic scaling and you end up with a completely different category of weather prediction that what we’re familiar with. Part of the existential crisis in weather forecasting behind closed doors right now is that this technology renders the NBM and much of the existing forecasting infrastructure obsolete, along with a lot of jobs devoted to developing and servicing the large global deterministic models. Lots of competing pressures and entrenched interests. -
January 2024 Weather in the PNW (Part I)
ajreich replied to Sunriver Snow Zone's topic in West of the Rockies
Without naming my sources I can confirm this It was obvious 5 years ago this would be the right answer. Combined with Spire’s remote sensing data from oceans and improved data ingestion pipelines, we’re on the cusp of a radical improvement in forecasting skill in the next 18 months. Once the training expands to include synthetic data, It’ll blow you all away how good it’s going to get and at what resolutions… -
NBM is quite an interesting product! Their weighting algorithm is moderately complex and dynamic, though does has some drawbacks as we saw yesterday. For those who don't know, NBM is an attempt by NOAA to create a super probabilistic forecast model that ingests output from all the models above and outputs forecast guidance for local offices that help them to gauge the relative odds of particular weather outcomes. The 'special sauce' is the post-processing, normalization and weighting that they do based on a number of factors, but most novelly, factoring in 'bias' or deviation of the forecast from historical observations (both short and long term) to adjust relative weights given to specific model outputs. Rather than static weights, it uses a 'learning' model that adjusts weights based on a number of dynamic factors for every data product release. Here's a somewhat dated visual of the weighting algorithm: (MAE is mean absolute error). I'm not sure about the latest version released last month, but previous NBM versions have used a 6-12 hour observation window for calculating MAEs and bias deltas (large recent deviations from observed will decrease model weighting), as well as factoring in model run consistency over the previous 7 days (larger run to run variance will necessarily decrease the model weight). So a couple of thoughts. First off, NBM is specifically designed to be a nationally consistent output, meaning that the entire thing runs for the entire country without regional or mesoscale differences. This clearly is a drawback when trying to predict fairly local effects like the gorge where few models are going to have the spatial resolution to accurately model small details like convergence zones. Second, the biasing model is trained based on historical deviations, rather than evaluated skill forecasting predicted weather patterns. In other words, the bias weight will factor in how a model has performed in the last 7 days, rather than 'how does the model perform for a given set of weather features.' There is nothing inherently wrong with this approach, and given the limitations with characterizing future weather projections it makes a lot of sense, but does have drawbacks. Specifically, with a pattern change like we saw yesterday: the forecast skill in the previous 5 days has little correlation to the skill forecasting the next 24 hours. So, why the lack of skill with the storm last night when some of the models did show big snow? I think a couple of obvious reasons. First off, most of the models flipped late to show big snow. This would have the effect of increasing the observed model variance (or uncertainty), leading to a lower weight in the NBM. Another factor was likely data ingestion. This is a national model, and for most of the country, input data is very good because weather patterns are moving over lots of land based sensors which can provide the core observation data used to weight the model. For us on the west coast, this is a bug not a feature, because the Pacific Ocean is a big data dead zone (largely we don't have much idea about the actual temp/pressure profiles except for a few buoys). So, when models flip at the last minute like they did last night, it's usually a data issue - better data has been used to initialize the models as weather features get closer to shore. But for rest of the country where weather data is consistent and good, last minute changes are an indication of model inconsistency rather than better data = poor model performance. Overall, I think NBM is a good product and provides a nice additional tool for NWS staff to tailor their local forecasts. But, like we saw last night, without understanding its limitations in specific scenarios (like we have with marginal snow events) the NBM can provide overly confident forecasts when in fact the confidence is decreasing. I think there's a lot of promise to this approach (and in fact NOAA is borrowing a lot from what commercial folks are already doing in big adaptive model development in finance and epidemiology): most obviously is another layer that weights model forecast based on prospective mesoscale patterns and allows for dynamic local weighting.
-
Pretty crazy day down here in NE PDX. Power flickering all morning, finally went out around 1:30. About 110k customers out of power as of 5 pm. Drove around and a good chunk of the east side is out of power from about 39th and I-84 out to 122nd and Division. Tons of street lights out, traffic is a mess. Given the backlog, I’m expecting we’ll be out of power a day or more. Luckily temps are in the low 40s, so not nearly as bad as last Thursday when we lost power in the east winds. After last years storms we put in a battery backup and so have heat and enough juice to watch some movies with the kids! We’ve had about 1.2 inches of rain. Not sure about wind since my station isn’t in a great place for wind.
-
Sitting at 30.5 in inner NE Portland since about midnight. Hoping we get scoured out soon with the first rain bands incoming.
-
Part I - January 2020 Weather Discussion for the PNW
ajreich replied to Timmy Supercell's topic in West of the Rockies
This is crazy town. The consistency with these snow numbers is unreal.